
10 Types of Risk Management Models You Should Know
Citrusx
Share
AI regulations are advancing faster than many financial institutions can adapt. As financial institutions deploy increasingly complex models, some built in-house, others acquired from third parties, they’re struggling to maintain their required visibility, explainability, and control. Opaque logic, shifting input data, and inconsistent validation practices create blind spots that traditional risk frameworks were never built to address. Without clear governance structures, even well-performing models become potential liabilities.
More organizations are recognizing that reality and preparing to respond. Instead of relying solely on generic model review processes, they’re beginning to invest in formalized functions specifically for AI risk management. In fact, 66% of firms that don’t currently have a dedicated AI risk management plan will establish one within the next one to four years. This shift reflects a growing consensus: AI requires its own approach to oversight.
Risk management models offer precisely that, structured, repeatable frameworks for evaluating AI systems against both internal governance standards and external regulations. Understanding these models helps close the gap between regulatory expectations and operational practices. Before choosing which to apply—or how to apply them—it’s worth taking a closer look at what they are and why they’ve become essential.
What Are Risk Management Models?
Risk management is the broader discipline focused on identifying, assessing, and addressing threats that could compromise business operations or regulatory standing. When it comes to AI, this includes everything from unintended bias and data drift to flawed assumptions and breakdowns in oversight. The complexity of modern AI systems—and especially those influencing financial decisions—requires targeted strategies built to evaluate risk at the system level.
Risk management models are structured frameworks that help organizations examine how models behave, under what conditions they might fail, and whether their outcomes align with established thresholds for safety and fairness. Some models emphasize process rigor; others are built to stress-test systems, flag instability, or expose areas of regulatory concern.
Used correctly, risk management models support auditability, reinforce compliance, and make it easier for technical and non-technical teams to collaborate around decisions that carry real risk. In environments where AI decisions must be explained, defended, and continuously validated, risk management models turn intent into action—and policy into practice.

Why Risk Management Models Matter for AI in Finance
Financial institutions rely on AI to make high-impact decisions like approving credit, detecting fraud, or assessing risk exposure. However, as these models become more complex, their inner workings become harder to interpret.
Legacy Controls Can’t Keep Up
Many AI/ML models are built using opaque techniques, trained on constantly shifting data, or developed by third parties with limited transparency into their design. These factors make traditional oversight approaches ineffective. Without structured governance, it becomes nearly impossible to evaluate whether models are performing as intended or exposing the organization to unacceptable risk.
Risk Models Enable Practical Oversight
Risk management models provide a way to restore clarity. They help map out areas of uncertainty, define what acceptable behavior looks like, and create traceable documentation for how models are evaluated over time. This is especially critical for validation and compliance teams, who need consistent frameworks for testing assumptions, reviewing outcomes, and justifying decisions to regulators and internal stakeholders.
Regulators Expect Structured Governance
That need for structure is reflected in how standards are evolving. ISO 42001, the first global AI management system standard, calls for formalized methods to identify, assess, and control risk across the AI lifecycle. While it doesn’t mandate specific tools, it emphasizes the importance of traceability, transparency, and accountable oversight—precisely the areas that risk management models are designed to support.

10 Types of Risk Management Models You Should Know
Embedding these ten frameworks into governance workflows allows organizations to replace reactive oversight with consistent practices that support responsible AI development:
1. The Three Lines Model
The Three Lines Model is a structural framework used to organize roles and responsibilities within risk management programs. It helps institutions establish a clear separation between those who build models, those who evaluate them, and those who audit the entire process.
The model is built around three distinct lines:
First Line - Operational teams who are responsible for designing, training, and deploying AI systems that manage risk directly during development and implementation.
Second Line - Independent risk and compliance functions that set policies, oversee compliance, and monitor exposure. They evaluate whether AI models meet internal standards, regulatory expectations, and ethical requirements.
Third Line - Internal audit, which provides independent assurance that both the first and second lines are functioning effectively. It assesses the overall governance framework, including controls, documentation, and accountability.
The Three Lines Model separates ownership from oversight, documents who are accountable at each stage, and ensures that no single team controls both model development and validation. For financial institutions, it reinforces internal governance and helps demonstrate that oversight processes are functioning as intended.

2. COSO Enterprise Risk Management (ERM) Framework
COSO ERM is a management framework that helps organizations think about risk in the context of strategy. It’s designed to make sure that risks of any type are identified, understood in context, and managed in line with business priorities.
What makes COSO ERM valuable is how it connects risk to business performance. It asks questions like:
What are we trying to achieve?
What could prevent us from getting there?
What level of risk are we willing to accept?
Who’s responsible for managing it?
This framework is often already in use by most financial institutions in areas like credit and operational risk. When applied to AI models, COSO ERM helps teams document how model behavior supports business goals, assess where it could break down, and ensure there are controls in place to intervene when needed.
3. NIST AI Risk Management Framework (AI RMF)
The NIST AI Risk Management Framework (AI RMF) is a U.S.-developed guideline created specifically for managing risks associated with AI systems. It focuses on making AI trustworthy by addressing key characteristics such as transparency, safety, robustness, and fairness. While voluntary, it’s quickly becoming a reference point for responsible AI practices, especially in highly regulated sectors like finance.
At the core of this risk management model is a four-part lifecycle approach:
Map the context in which AI is being used, including its intended purpose and potential impacts.
Measure the risks, limitations, and performance characteristics of the system.
Manage risks through mitigations, oversight mechanisms, and response planning.
Govern the entire lifecycle with clear policies, roles, and accountability.
This structure helps institutions identify where AI systems could introduce risk and offers a standardized way to address it. The framework also complements global standards like ISO 42001 and supports the level of traceability and control that upcoming regulations are expected to require. Citrusˣ supports this lifecycle approach by providing built-in tools for model documentation, performance tracking, and explainability.

4. RACI Model for Model Risk Accountability
The RACI model offers financial institutions a lightweight, structured way to reduce ambiguity and enforce accountability as models move through the lifecycle. It helps manage model risk by ensuring accountability is distributed, conflicts of interest are avoided, and oversight gaps are less likely to emerge.
RACI works by assigning each task or decision to one or more roles based on four categories:
Responsible: The person or team doing the work
Accountable: The individual is ultimately answerable for the outcome
Consulted: Stakeholders whose input is required
Informed: Stakeholders who need to be kept in the loop
RACI is beneficial in environments where developers, business leads, compliance officers, and risk managers all contribute to decision-making. This structure also improves traceability. When roles are clearly assigned, it’s easier to document who made or approved critical decisions, which supports both internal governance policies and external audit requirements.
5. Risk Heat Maps / Risk Matrices
Risk heat maps and matrices help institutions identify which AI models carry the highest risk and require the most oversight. By visualizing risk based on likelihood and impact, they make it easier to focus governance efforts where failure would be both probable and costly.
The format of heatmaps is simple but effective:
Likelihood reflects the chance of failure or unintended outcomes.
Impact measures the potential severity of those outcomes.
Plotted together, they highlight which models need greater scrutiny.
High-risk models identified through these tools can be flagged for additional oversight, governance review, or escalation. This helps prevent critical issues from being overlooked, especially in large portfolios with models at different stages of maturity.
Heat maps also create a structured, explainable way for compliance teams to assess and document risk. When presented during audits or regulatory reviews, they help demonstrate that model oversight is active and grounded in a consistent, risk-based methodology.

6. Model Validation Frameworks (SR 11-7)
Model validation frameworks like SR 11-7 are essential for managing AI model risk. They ensure that models perform as intended, are used appropriately, and remain stable over time, such as when conditions, inputs, or business contexts change. These frameworks also help financial institutions meet regulatory expectations by enforcing independent review and formal governance around model development and deployment.
At the core of most validation frameworks is a three-part process:
Performance testing to evaluate accuracy, robustness, and generalizability.
Assumption review to assess whether model logic, inputs, and parameters are sound.
Limitations analysis to identify where the model may fail or produce unreliable outputs.
Strong frameworks also include supporting governance elements:
Ongoing monitoring to catch drift or performance degradation.
Documentation standards to track changes, ownership, and usage.
Change control to manage when and how models are updated or replaced.
U.S. regulators—including the Federal Reserve, which issued SR 11-7, and the Office of the Comptroller of the Currency (OCC)—require financial institutions to independently validate all material models and maintain thorough documentation of testing, findings, and corrective actions. Citrusˣ supports this process by tracking model behavior in real time, flagging performance issues immediately, and generating explainability reports that are audit-ready, reducing manual effort while reinforcing compliance.
7. Scenario Analysis and Stress Testing Models
Scenario analysis and stress testing are risk management models used to assess how AI systems perform under adverse conditions. They help institutions identify weak points in model behavior that may only emerge during market stress, regulatory shifts, or other disruptive events. They give teams the ability to plan and respond before those risks become real-world failures.
These models work by simulating conditions that fall outside the model’s baseline assumptions. A typical stress test might involve changes in:
Economic indicators, such as unemployment or inflation
Policy shifts, like interest rate changes or new lending rules
Behavioral patterns, such as sudden spikes in default rates or transaction anomalies
By comparing how model outputs respond to these scenarios, teams can uncover where predictions become unstable, accuracy drops, or outcomes deviate from expected behavior. This is especially important for models exposed to external volatility, such as those used in credit risk, capital forecasting, fraud detection, AML, or liquidity planning, where performance under stress directly affects financial and regulatory outcomes.
These practices are also a compliance expectation. U.S. regulators, including the Federal Reserve and the OCC, require stress testing for high-impact models and expect institutions to document how those models respond to extreme but plausible scenarios.
The same logic applies to AI systems in critical infrastructure, like smart water networks, that must remain stable during equipment failures, weather events, or demand surges. Scenario analysis helps ensure these systems can continue operating safely, even under stress.

8. MAS Information Paper on AI Model Risk Management
Spreading in use across Asia, the MAS Information Paper provides a regulator-endorsed risk management model specifically for AI. Published in December 2024 by the Monetary Authority of Singapore (MAS), it outlines best practices for managing high-risk and third-party AI models across governance, validation, and ongoing monitoring.
Key practices include:
Defined accountability for model ownership and review
Independent validation, separate from development teams
Continuous monitoring for performance, fairness, and data quality
What sets the MAS paper apart is its focus on real-world implementation. It addresses emerging risks unique to AI, such as adaptive learning and vendor opacity, and grounds its guidance in examples from financial institutions. As a regulator-authored benchmark, it offers teams a practical foundation for building AI governance frameworks that are both supervisory-aligned and deployment-ready.
9. ISO 42001: AI Management Systems
ISO 42001 is the original international standard for managing AI risk at the organizational level. Published in 2023, it provides a formal governance framework that helps institutions identify risks, assess impact, implement controls, and continuously monitor AI systems in production.
The standard outlines how to:
Integrate AI oversight into enterprise risk management.
Define roles and accountability for AI development and use.
Establish controls for risk monitoring, auditability, and continuous improvement.
Unlike voluntary guidelines, ISO 42001 is meant for certification. That means organizations can be independently assessed and validated against its requirements, making it valuable for institutions managing high-risk AI systems or working with third-party vendors.
ISO 42001 also aligns with other ISO frameworks like ISO 27001 (information security) and ISO 9001 (quality management), so it’s easier to embed AI governance into existing compliance structures. It offers a practical, internationally recognized risk management model for financial institutions to operationalize oversight and build trust, both internally and across partner ecosystems.
The same applies to high-impact AI systems outside of finance, such as materials informatics platforms used in pharmaceutical or industrial R&D. These platforms often use evolving models and ingest data from varied sources, which makes structured oversight and lifecycle governance essential for maintaining traceability and meeting regulatory expectations.
10. EU Artificial Intelligence Act (EU AI Act)
Finally, the EU AI Act is a binding regulatory framework that formalizes AI risk management across the European Union. Entered into force in August 2024 (with high-risk requirements beginning to apply in 2026), the act requires organizations to classify AI systems by risk level and imposes strict obligations on those deemed high-risk, such as credit scoring, fraud detection, and biometric identification.
The regulation mandates that high-risk systems must meet specific requirements, including:
Risk and impact assessments before deployment
Transparent documentation of model purpose, behavior, and limitations
Ongoing monitoring and human oversight throughout the model lifecycle
Unlike advisory frameworks, the EU AI Act carries legal consequences for non-compliance. For financial institutions operating in or serving the EU, it defines how AI models must be evaluated, governed, and audited in practice.
As a risk management model, the EU AI Act forces teams to move beyond technical performance alone and establish structured governance from development through deployment. Citrusˣ supports EU AI Act compliance by enabling end-to-end risk classification, model documentation, and real-time monitoring for high-risk systems.
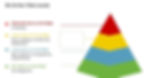
How Citrusˣ Operationalizes Risk Management Models
AI adoption in financial services is accelerating—and with it, there is a need for structured oversight. These risk management models offer financial institutions a way to evaluate, monitor, and govern AI systems effectively while aligning with increasing regulatory expectations.
Citrusˣ enables financial institutions to operationalize these models by replacing manual oversight and fragmented tools with a single system for AI validation, monitoring, compliance tracking, and explainability. The platform meets the demands of evolving AI regulations while giving teams deeper visibility and control across the full model lifecycle.
Book a demo today to discover how Citrusˣ can help your team bring structure and accountability to AI model risk management.
Share
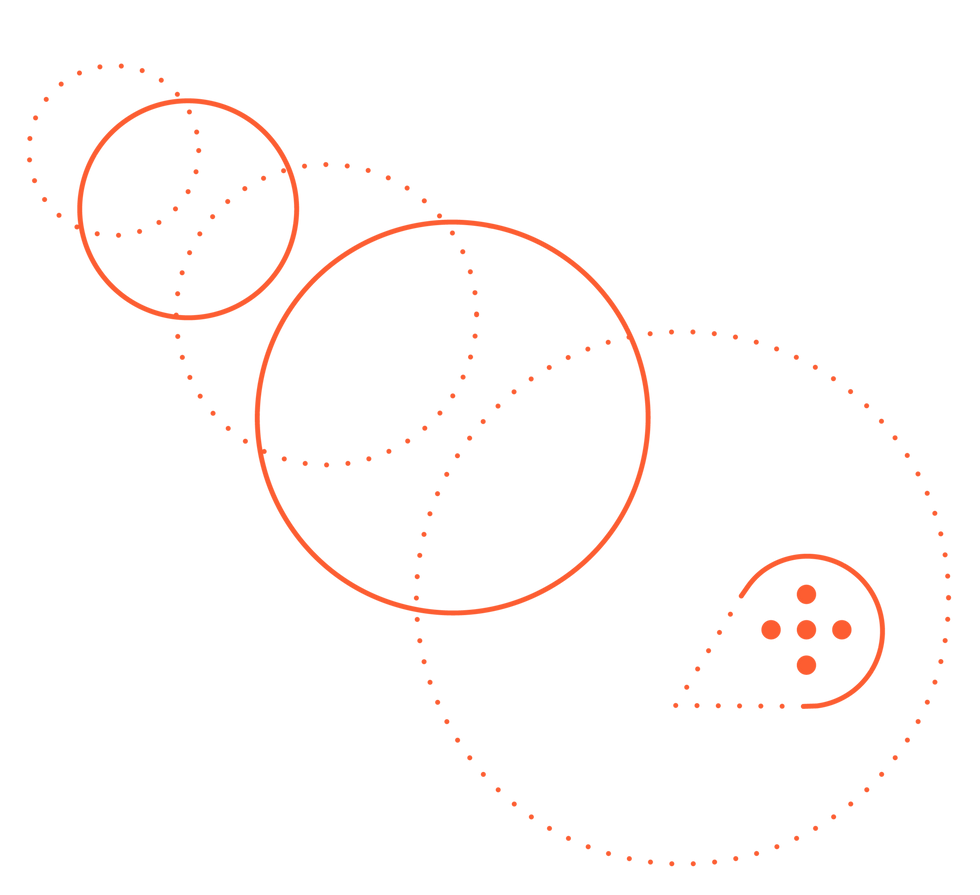