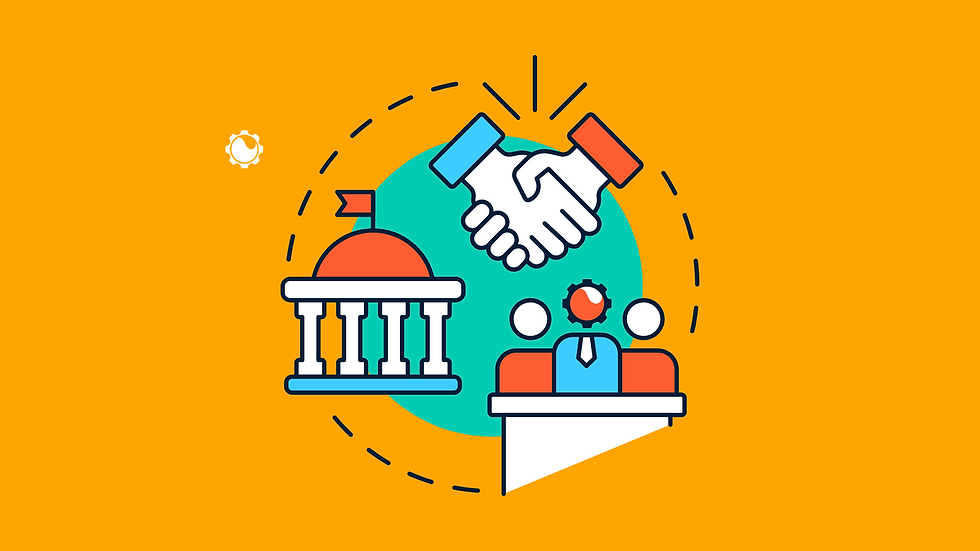
7 Key Elements of AI Ethics and Governance
Citrusx
Share
What happens when an AI model generates a decision your institution can't justify?
Consider this scenario: A credit risk model performs well in testing. An online bank deploys it to support lending decisions. But months later, a series of customer complaints triggers a compliance review. The team can't explain how the model arrived at specific outcomes, and its performance hasn't been monitored since it went live. In review, it becomes clear that no one is responsible for validating whether the system still aligns with policy. The model hasn't failed—but the bank's AI governance has.
With AI, ML, and GenAI tools becoming more embedded in high-stakes financial workflows, oversight is no longer optional. Regulators are increasingly scrutinizing how AI models are governed. They expect institutions to show that a model performs as intended, and that it's actively managed with the appropriate controls in place. Research indicates that only 21% of executives say their organization's AI governance practices are systemic or mature. This disconnect between growing regulatory demands and limited internal governance capability presents legitimate operational and reputational risks.
AI ethics and governance address these risks directly. For financial institutions using AI to support high-stakes decisions, AI ethics and governance offer a way to manage these systems with the same level of control expected across the rest of the organization. Let's take a closer look at the foundational elements that make this possible.
What Is AI Ethics and Governance, and Why Does It Matter?
AI ethics and governance are the principles and processes that ensure AI systems are designed, deployed, and managed responsibly. They define:
How AI, ML, and GenAI models are approved.
How model performance is evaluated.
How accountability is maintained once systems are in use.
In regulated industries, AI ethics and governance provide the structure needed to ensure models operate responsibly over time. It includes oversight of risk, alignment with compliance standards, and adherence to business policy, while also upholding ethical principles in how decisions are made and applied.

The need for strong AI ethics and governance is growing as AI becomes embedded in critical financial workflows. Without robust governance, even well-performing models can cause outcomes that are difficult to explain, justify, or defend. AI ethics and governance give institutions the ability to detect issues early, enforce accountability, and demonstrate to regulators that systems are under active control.
The responsibility for AI ethics and governance spans organizations to include:
Technical leaders responsible for building and deploying models that meet ethical, performance, and regulatory requirements.
Compliance teams tasked with overseeing model risk, documentation, and alignment with evolving standards.
Business stakeholders accountable for ensuring that AI-driven decisions support institutional goals and customer outcomes while maintaining trust.
When governance is implemented effectively, it builds trust across the board. It strengthens internal coordination, satisfies external oversight, and ensures that AI is developed and deployed in line with institutional values and public expectations.
7 Essential Elements of AI Ethics and Governance
Here are the foundational elements of AI ethics and governance that financial institutions need to build trustworthy, compliant, and high-performing AI systems:
1. Transparency and Explainability
Transparency means knowing how an AI system was developed and how it operates in production. Explainability is the ability to clearly describe how a model arrives at specific decisions using language and evidence others can review and evaluate.
These elements are essential for ethical governance. Institutions need to trace how decisions are made, determine whether those decisions align with policy, and intervene when outcomes fall outside acceptable bounds. Without transparency and explainability, oversight becomes impossible, and accountability disappears.
From a regulatory perspective, standards like SR 11-7 and Article 13 of the EU AI Act require institutions to document how models work, show how decisions align with policy, and ensure that humans can review the logic behind those decisions. Ethical AI demands that decisions are accurate and defensible.
2. Fairness and Bias Mitigation
Fairness means ensuring that AI systems do not create unjustified differences in how people are treated. In financial services, this applies to models that determine credit eligibility, flag transactions for fraud, or influence customer interactions and access.
If a model consistently produces outcomes that disadvantage certain populations, it becomes both a governance risk and an ethical failure. These disparities can undermine trust and trigger compliance violations, even when the model is technically accurate.
Institutions address these issues through the bias mitigation process, which includes:
Applying fairness metrics to identify discrepancies across groups
Rebalancing training data to address imbalances in representation
Adjusting decision thresholds to meet fairness objectives
Using manual review for high-risk decisions where automation may introduce bias
These practices are central to AI ethics and governance because they ensure that fairness is tested, reviewed, and documented.

3. Accountability and Stakeholder Alignment
Accountability means knowing who is responsible for every part of an AI system, from design to deployment to monitoring. It ensures that every decision and every risk assessment can be traced to someone empowered to manage it.
Without that clarity, gaps form between teams. For example, one group might build an AI model to power a wastewater management solution, another validates it, and a third deploys it—but without shared visibility or coordination. This kind of breakdown is a governance failure.
Stakeholder alignment requires agreement on who is responsible for functions like performance, documentation, and oversight. It also depends on sustained collaboration between technical, risk, compliance, and business teams throughout the model's lifecycle.
Strong model governance makes this operational. It defines who approves what, how decisions are escalated, and where accountability lives across teams. When this structure is in place, risk is reduced, but if it's missing, even good models can become dangerous.
4. Proactive Monitoring and Oversight
Proactive model monitoring and oversight are the systems that ensure AI behaves as intended upon deployment. These processes track how models perform in production and evaluate how outputs evolve. Most importantly, they allow institutions to respond when results begin to drift from policy, performance, or ethical expectations.
Two types of drift are important in this context:
Data drift occurs when the inputs change, such as when customer behavior shifts, or a source system is updated.
Ethical drift refers to gradual changes in model behavior that move it away from approved ethical standards. It can occur even when performance metrics look stable because the model's decisions no longer align with fairness, transparency, or policy intent.
Both forms of drift carry risk. If they go undetected, institutions lose visibility into the systems they rely on. That can lead to compliance failures, customer harm, or reputational damage.
Proactive monitoring and oversight help prevent those outcomes by giving teams continuous insight into how the model is behaving and whether its decisions are still acceptable. Governance defines what to monitor, who is responsible for review, and how issues are escalated when thresholds are breached.

5. Data Governance and Quality
Ethical AI starts with reliable data via data governance and quality:
Data governance is the overall framework of policies, processes, roles, and standards an organization implements to manage its data assets effectively throughout its entire lifecycle.
Data quality ensures that the information used to train and operate models is accurate, complete, and appropriate for the task.
Building trust in AI begins with data confidence. Institutions must understand where their data comes from, how it has changed over time, and whether it reflects the populations the model will affect. That includes validating inputs, documenting lineage, and checking for imbalances before training begins.
When data is flawed, it undermines even the most sophisticated models. Biases in historical records or mislabeled fields can lead to decisions that are challenging to justify and misaligned with institutional policy. These issues raise ethical concerns, especially when model outcomes affect financial access or regulatory exposure.
Governance ensures standards are upheld by assigning responsibility for data integrity. Reviews are enforced at key points in the development process, and controls are put in place to prevent low-quality inputs from reaching production. Without these safeguards, there is no ethical foundation for AI.
6. Regulatory Awareness and Readiness
AI ethics and governance are increasingly shaped by regulation. Regulatory readiness means designing AI systems that align with legal expectations from the start rather than retrofitting controls after deployment. It is how institutions ensure that their models can stand up to scrutiny.
Many AI regulations are now in effect, including:
The EU AI Act introduces formal risk categories, documentation rules, and oversight requirements.
ISO 42001 sets governance standards for managing AI across the enterprise.
In the U.S., financial regulators are applying existing model risk principles from SR 11-7 to more complex AI systems.
Connecting these external requirements to internal practices is the role of governance. It creates documentation, assigns accountability, and embeds oversight throughout the model lifecycle. Financial institutions that apply these principles early reduce the friction of audits and avoid the risk of retroactive remediation. Being prepared for regulation is a sign that ethical governance is functioning.
7. Building a Culture of Ethical AI
An ethical AI culture shows that teams understand their responsibility and take it seriously. It is about building systems with clarity and care, knowing that the impact of each decision extends beyond the model itself. Without this mindset, even well-designed governance frameworks can break down—and when ethical responsibility is not shared, it gets ignored.
Organizations like financial services companies and generative AI startups that lead on this front invest in a culture of ethical AI. It includes ethics councils with cross-functional participation, regular training for model developers and risk reviewers, and internal forums for raising concerns. It also means involving non-technical voices in design and validation, especially when model decisions affect people's lives.
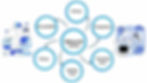
Cultural values become operational standards through governance. It defines how ethical concerns are handled, how responsibilities are assigned, and how ethical thinking becomes part of the development process. A culture of ethical AI protects the integrity of the entire system.
How Citrusˣ Helps Implement AI Ethics and Governance
Implementing AI ethics and governance across your organizations and throughout your model's lifecycle is best performed with the help of a solution like Citrusˣ. It's an end-to-end AI and LLM validation and risk management platform designed to help financial services organizations build and maintain trustworthy models.
The platform equips teams with tools to validate models, monitor performance, ensure explainability, mitigate bias, and demonstrate compliance with evolving regulatory standards.
Each of the seven key elements of AI ethics and governance is directly supported by Citrusˣ's core capabilities:
Transparency and Explainability: The platform creates model documentation and visual explanations that clarify how decisions are made, enabling faster reviews and more transparent communication with internal stakeholders and regulators.
Fairness and Bias Mitigation: It includes built-in fairness metrics, automated bias detection, and configuration options that support targeted remediation across model inputs and outputs.
Accountability and Stakeholder Alignment: Role-based workflows, integrated review paths, and approval tracking ensure that responsibilities are clearly defined and traceable across risk, compliance, and technical teams.
Proactive Monitoring and Oversight: Real-time performance monitoring, drift detection, and alerts allow teams to identify issues early and act before models fall out of policy or compliance.
Data Governance and Quality: Checks for missing data, verifies alignment between training and validation datasets, and monitors for data drift to support auditability and consistent model behavior.
Regulatory Awareness and Readiness: The platform generates audit-ready documentation, automates governance workflows, and supports compliance with regulatory frameworks.
Culture of Ethical AI: By embedding governance into everyday model operations, it promotes shared responsibility and creates space for ethical review throughout the AI lifecycle.

Responsible AI Starts with the Right Foundation
The integration of AI into critical financial workflows makes AI ethics and governance essential for ensuring responsible and compliant operations. Focusing on these seven elements empowers an organization's governance, ensuring AI models drive ethical outcomes that align with business objectives and regulatory demands.
This is where Citrusˣ offers a critical operational solution. It directly supports teams in accelerating validation, streamlining compliance, improving collaboration, and reducing governance complexity. Designed for real-world implementation, it enables financial institutions to scale their AI deployment with both control and confidence.
Book a Citrusˣ demo today to discover how it facilitates robust AI ethics and governance in your organization.
Share
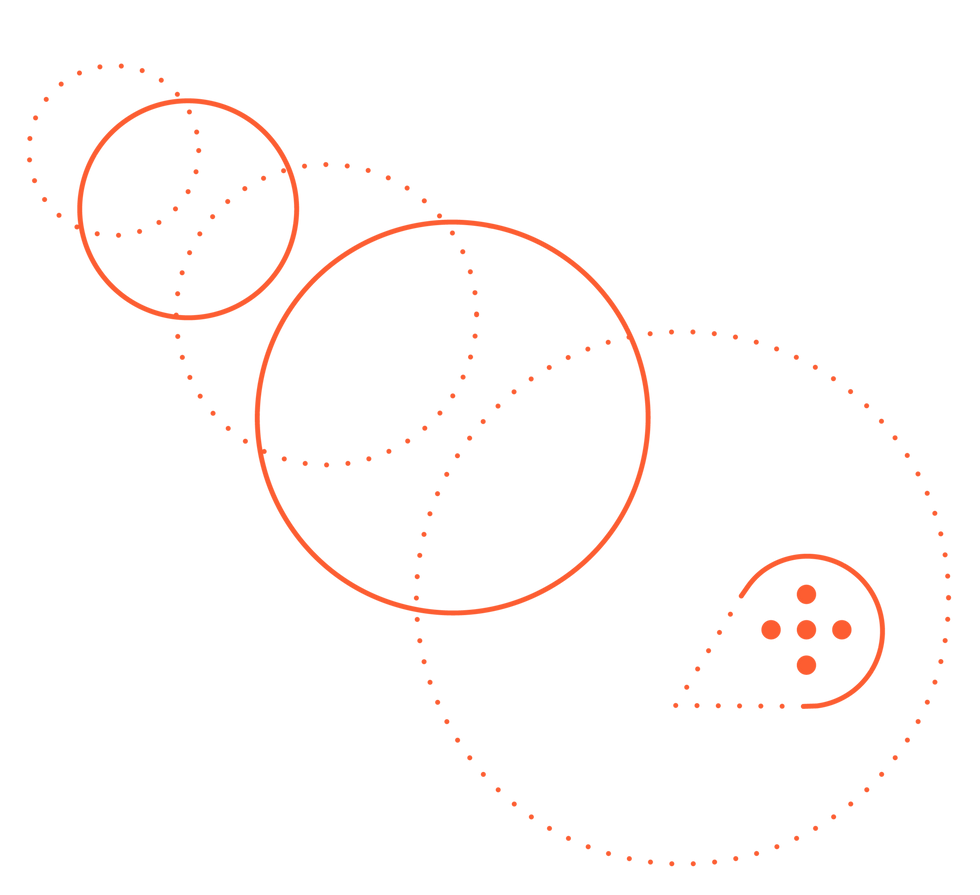